VEIL - Alice Hein & Lukas Meier
Summarized by Robin Preiß
Highlights of the VEIL
The Ethical Innovation Hub's ninth Virtual Ethical Innovation Lecture featured Alice Hein from the Technical University of Munich, where she works as a research assistant at the chair of data processing, held by Prof. Dr.-Ing. Klaus Diepold, and Dr. Lukas Meier from University of Cambridge’s faculty of philosophy, where he works as a junior research fellow specializing in bioethics and the philosophy of mind.
In their talk, Alice Hein and Lukas Meier presented first insights of their research project “METHAD”. The project focused on devising an algorithm to aid in the ethical decision making in clinical contexts. The algorithm is based on real life decisions and designed to cope with numerous ethical questions that arise frequently. Its aim is to support ethics committees, potentially taking over the host of more standard issues, while opening up time to allow the committees to concentrate on the more involved dilemmas.
Introduction and Approach
Lukas Meier started the presentation, outlining the aim of the project. The algorithm should support ethical decision making in the clinic, addressing dilemmas ranging from, e.g., decisions at the beginning and the end of life, the patient’s right to refuse or request specific treatments or confidentiality issues. To make this tangible, Meier presented three example questions which frequently occur in clinical contexts:
- Should doctors continue treating a child who still has a small chance of long-term survival against her will?
- Should one carry out a procedure that has adverse medical effects when the patient insists on receiving the treatment?
- Should medical personnel try to save a patient’s life following a suicide attempt when she had signed a do-not-resuscitate order many years ago?
Those questions are usually discussed in clinical ethics committees, in order to give proper advice and reach a recommendation for action. These committees are confronted with a rising number of cases, which can be overwhelming, especially in urgent situations. Moreover, some emergency situations may exceed the human capacity to factor in all the relevant data in a short time frame. It is argued that decision support may facilitate more consistent ethical recommendations, especially when based on large data sets.
Hence, to support ethical decision making, it is the goal to create an algorithm which can make faster decisions than humans and might also be more accurate.
Ethical Foundation of the Algorithm
Lukas Meier discussed an investigation into consequentialist, deontological and virtue ethics to be considered as foundational frameworks for the algorithm. Following a single ethical framework appeared problematic and empirical research is presented to show that there is no real consent how to act. Therefore, rather than embedding foundational ethical frameworks, the principles of biomedical ethics due to Beauchamp and Childress (1979) has been adopted as the de facto consensus in applied medical ethics. The four principles are:
- beneficence,
- non-maleficence,
- respect for autonomy, and
- justice in the distribution of healthcare.
To cope with the ethical problems the research team tried to integrate the four principles in the algorithm by collecting premises which play a role in clinical case discussions. They collected cases based on which the algorithm could then determine which should be the desired outcome, via regarding recorded decisions of clinical ethics committees.
Technical approach
Subsequently, the technical aspects were presented by Alice Hein. Fuzzy cognitive maps were chosen due to their similarity to causal graphs and the ability to explore the decision space. At the first the approach is focused on the principles of autonomy, beneficence and non-maleficence, while the principle of justice will be addressed later. The method allows for an intuitive visualization that is easy to extend. Furthermore, fuzzy cognitive maps does not require as large amounts of data for training when compared to other machine learning approaches. Being able to rely on relatively few cases was a necessity that arose due to privacy issues.
The weights that determine the influencing factors of proposed decisions can be trained with a genetic algorithm. The data for the training is grounded in a survey of ethicists deciding on textbook cases. After training, it was investigated to what extent the predictions correlate with the assessments of the ethicists. On a normalized scale, where 0 stands for “don’t intervene” and 1 for “definitely intervene”, the performance of the algorithm showed an average deviation from the solutions specified by the ethicists of 0.11 on the training set and 0.23 on the test set. Furthermore, the average agreement with the ethicists was 92% with respect to the cases in the training set and 75% of cases in the test set.
Example Case 1
The patient is 15 year old, suffering from leukaemia for 9 years and chemotherapy would yield 1 additional year of life but also reduces the quality of life. The patient then prioritizes quality of life over an extension and wants to die at home. The question asked is if an intervention should be attempted.
Example Case 2
The second example showed a suicide attempt where the patient’s advance directive was, “do not resuscitate” and the surrogate decision maker says “do resuscitate”. This example showed a conflict which could not be resolved even after 100 iterations of running the decision algorithm. Instead, the algorithm’s output showed oscillatory behavior, despite the fact that the patient advance directive has more influence.
Frontend and Solution
In the further course of the project, an app was developed with different ethical parameters for specific cases. The aim of the app is to show to what extent the prediction is for or against an intervention.
Q&A-Session
Questions being raised (and addressed) in the aftermath of the talk revolved around different views of the ethicists regarding the disagreement for specific cases, the potential demand of clinicians for such tools, other ethical side effects for the potential user and the framing of potential mismatches in the scales.
Literature
Beauchamp, Tom L. and James F. Childress (1979). Principles of Biomedical Ethics. New York: Oxford University Press
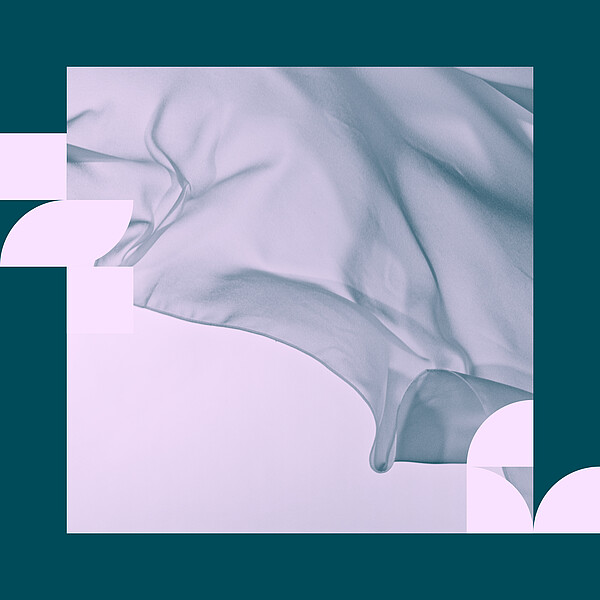